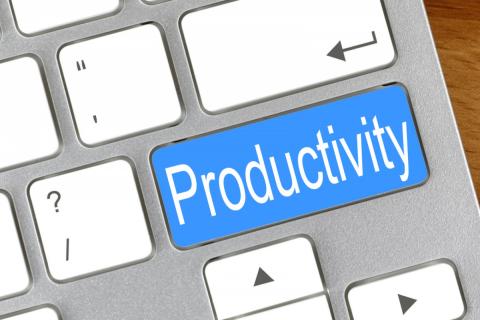
Since generative artificial intelligence captured popular imagination a year or two ago, I've heard a lot about "supercharging your productivity with AI" (Microsoft 365), "re-igniting productivity and inclusive income growth" (McKinsey) and the like.
The degree to which computing technology increases productivity is actually a long-standing question in economics, going at least as far back as the 1980s, when economist Robert Solow famously observed "we can see computers everywhere except in the productivity statistics." More recently, the OECD's Digitalisation and Productivity report (2019) observed
Digital technologies are transforming our economies and seem to offer a vast potential to enhance the productivity of firms. However, despite ongoing digitalisation, productivity growth has declined sharply across OECD countries over the past decades.
To be specific, economists define productivity as the ratio of economic output to input resources (labour, raw materials, and so on). Labour productivity is the economic output from an hour of work and total factor productivity is the economic output from all input resources. The higher the productivity of an organisation (a "firm" in economics jargon) or a country, the more goods and services that organisation or country can produce with same amount of work and raw materials. Productivity growth is the increase in productivity from year to year due to technological advances, improved work processes, and so on. Economists and political leaders like to see high productivity growth because it increases the number and quality of goods and services available.
Numerous academic studies have tried to answer to the question of whether and how computing technology affects productivity growth, most recently surveyed by Khuong Vu and colleagues (2020) and Stefan Schweikl and Robert Obermaier (2020).
Both reviews agree that studies prior to the 1990s show little impact of computing technology on productivity growth within firms, but they differ slightly on what happened from about 2005 or so. Vu and colleagues say that productivity growth due to computing has increased since the mid-1990s, and are confident enough to say that no more studies are necessary to show that computing has a positive impact on productivity growth; research should now focus on the mechanisms by which this impact is made. Schweikl and Obermaier agree that productivity growth due to computing increased significantly from the mid-1990s, but agree with the OECD report that productivity growth has decelerated since the mid-2000s. These two interpretations can be reconciled by observing that Vu and colleagues treat 1997-2017 as a single period, which includes the high-impact 1997-2007 period, meaning the whole 1997-2017 period shows greater impact than the 1977-1997 period. I will therefore accept Schweikl and Obermaier's characterisation as being broadly correct.
Schwiekl and Obermaier say that few studies exist that might explain the deceleration of productivity growth observed between the mid-2000s and the time of their study, but identify four broad explanations proposed for the Solow Paradox of the 1980s:
- the length of time required for organisations to learn to apply computing technology effectively;
- difficulties in measuring either input or output factors;
- exaggerated expectations (that is, computing technology simply isn't as effective as its proponents supposed); and
- lack of complementary investment required to effectively deploy and use computing technology.
The OECD report appears to be in the last camp. It argues that productivity gains have varied widely from firm to firm, concluding that "firms having better access to key technical, managerial and organisational skills have benefitted more than other firms". The report therefore recommends training in skills that would improve use of computing technology and development of strategies to better "reallocate" capital and workers to more productive firms.
None of the views imply that the mere existence of computers, artificial intelligence, or anything else automatically leads to explosions in productivity. Most obviously, the "learning curve" and "complementary investments" explanations suppose that technology needs to be accompanied with training, experimentation, business transformations and the like to make effective use of the new technology. The "exaggerated expectations" explanation supposes that the productivity growth we're getting now is about as good as we can reasonably expect (including whatever training, etc., we might do). The "mismeasurement" explanation supposes that productivity growth might exist but that it can't be measured by the tools that economists currently have—but if we can't measure it, how can we say that it exists?
We can't yet know whether artificial intelligence or any other technology will bring back productivity growth like that of the late 1990s and early 2000s. It should be said that even low productivity growth is better than no productivity growth at all (assuming that what you're producing is a good thing)—it just means that productivity is merely "improving" rather than "supercharging".
References
Organisation for Economic Cooperation and Development (2019). Digitalisation and Productivity: A Story of Complementarities.
Stefan Schweikl and Robert Obermaier (2020). Lessons from Three Decades of IT Productivity Research: Towards a Better Understanding of IT-induced Productivity Effects. Management Review Quarterly 70, pages 461–507.
Khuong Vu, Payam Hanafizadeh and Erik Bohlin (2020). ICT as a Driver of Economic Growth: A Survey of the Literature and Directions for Future Research. Telecommunication Policy 44(2), article 101912.